
Return tf.cast(image, tf.float32) / 255., label """Normalizes images: uint8 -> float32.""" (ds_train, ds_test), ds_info = tfds.load( Print("Num GPUs Available: ", len(tf._physical_devices('GPU')))įrom import disable_eager_execution
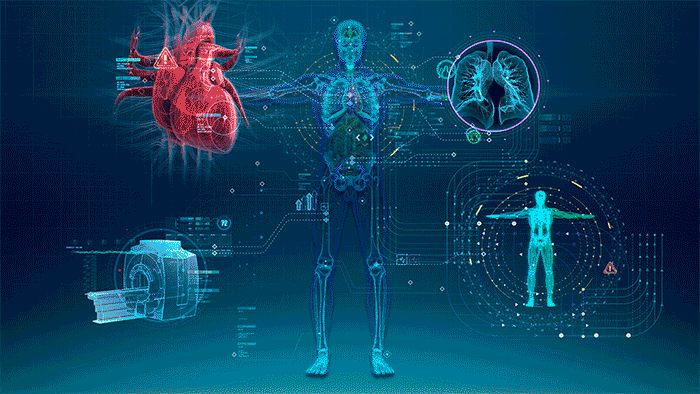
I would appreciate very much any help from Apple support or the developers community.
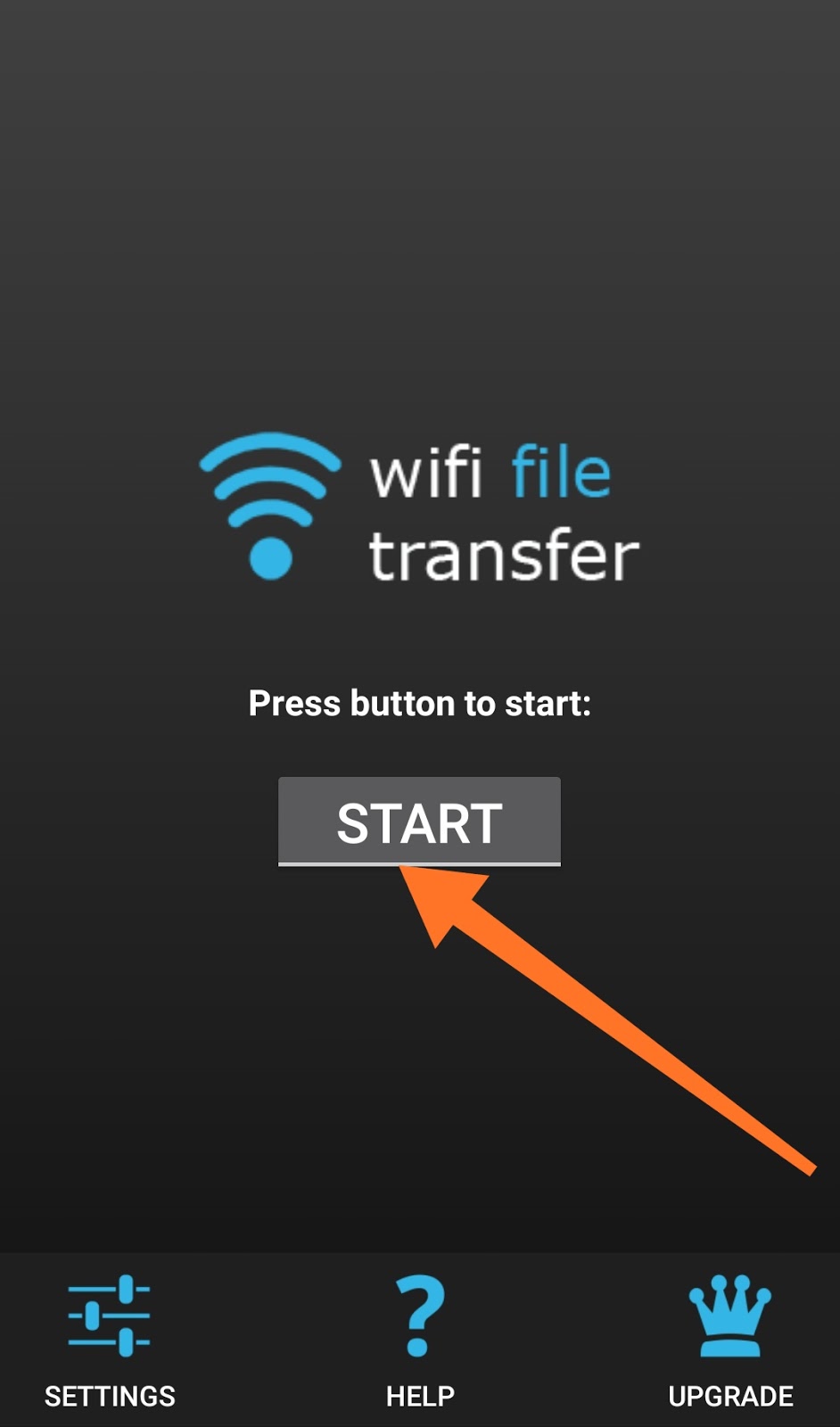
We have more than 50 data scientists in our company and I am leading a research on CoreML and the adoption of the new MacBook Pro as a standard platform to our developers. As a remedy I am now running the same code on Anaconda (Rosetta) and it is taking 50% more time. I have formatted the MacBook several times, followed the instructions on and the problem persists.
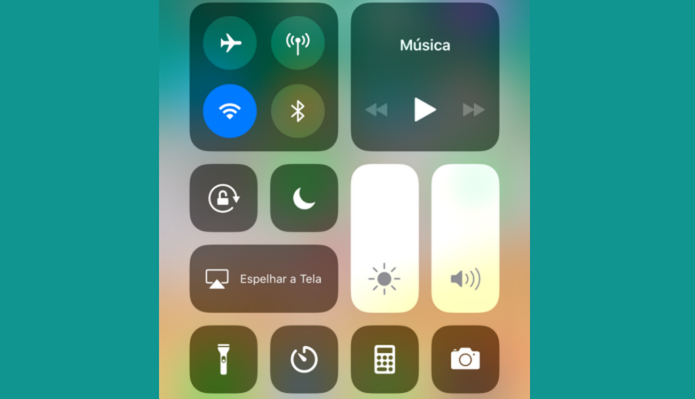
I'd been successfully running M1 native Python code on a MacBook Pro (13-inch, M1, 2020) using Jupyter Notebook, but since the notebook kernel dies as soon as the M1 CPU is used intensively. Please, I need help to run M1 native Python again!
